In this chapter, we propose a novel video alignment method that works directly on time domain based on slice matching. Furthermore, the proposed algorithm deals with sequences recorded from independently moving cameras whose trajectories can be more or less coincident or parallel. The key idea of the algorithm is to exploit the analogy between image matching and slice matching. As image matches are processed to obtain the geometric transformation between images, slice matching provides temporal correspondences that can be processed towards temporal mapping. Although image matching via retrieval can also lead to synchronization, by slice matching we work directly on time domain. These putative temporal matches are viewed as samples of a frame correspondence pdf described by a Gaussian mixture model (GMM). The GMM parameters are learnt through a Maximum Likelihood Estimation, that also permits us to process new types of sequences, like indoor sequences, without manual parameter tuning. We then formulate the temporal mapping estimation as a maximum a posteriori inference problem based on probabilities extracted by the learnt probability density function (pdf), in contrast to the previous chapters and past works where they are estimated empirically. Instead of spatially registering corresponding single frames using Lucas-Kanade algorithm, we use the recently proposed ECC image alignment algorithm that offers robustness to appearance variation.
|
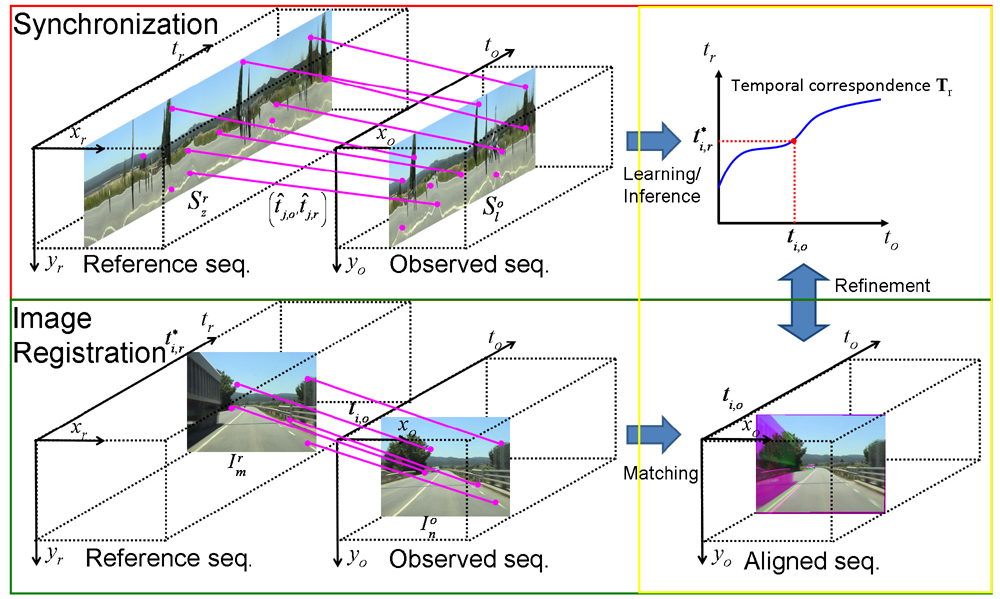
|