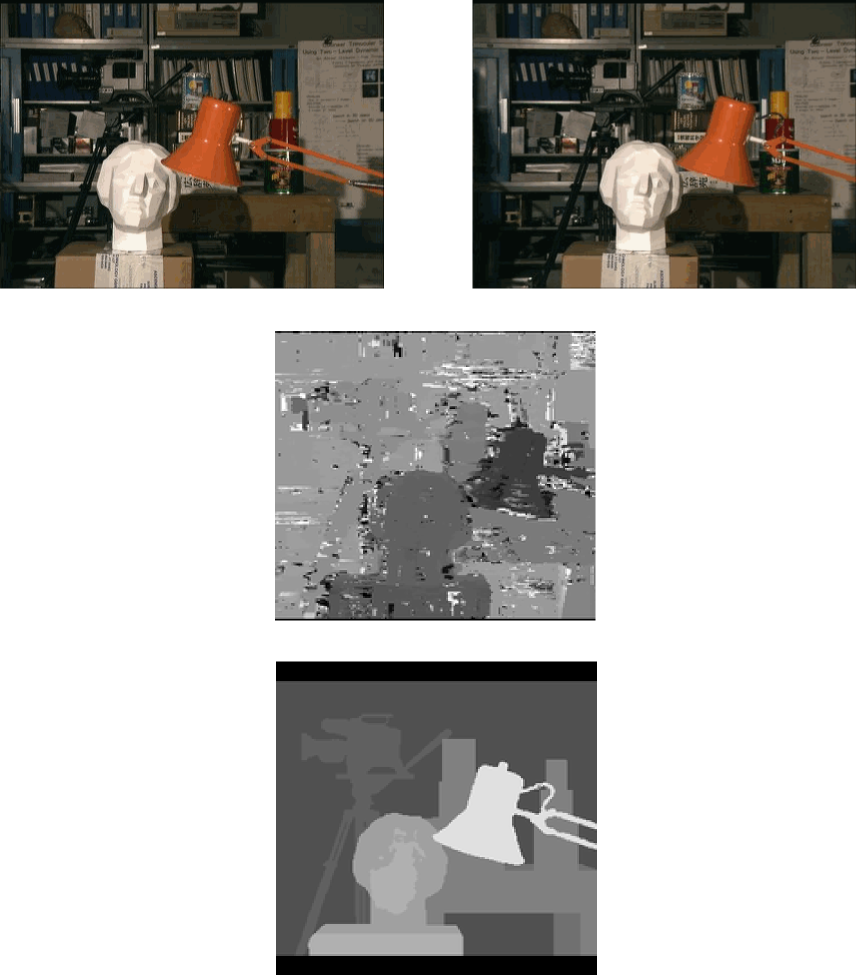
Abstract
This course introduces elements of computer vision and optimization techniques. These are at the heart of cutting-edge machine vision and consumer hard- and software.Format
- Lectures: April 7-11 2014, 09:30-12:30 in the HCI, Speyerer Str. 6, second floor.
- Exercises: April 7-11 2014, 13:30-18:00 also in the HCI, 2nd floor.
Contents
Monday:- some applications of computer vision
- stereo vision (epipolar geometry ,patch similarity measures ,cost volume)
- cost volume filtering as approximate optimization strategy
- Markov random fields / undirected graphical models
- example: Potts model for dense stereo
- ILP formulation
- branch & bound
- Kappes benchmark
- OpenGM
- exact inference on trees: belief propagation / dynamic programming
- example for stereo vision: optimization for individual scanlines
- LP relaxations
- dual decomposition
- example: coupled scanline optimization for dense stereo
- simplex algorithm
- interior point algorithm
Formalities
This course accounts for 2 CP and is eligible as part of the new Physics Vertiefungsbereich "Computational Physics (MVX)".Prerequisites
Standard algebra and calculus. Working knowledge of some programming language.Literature
- Algorithm Design Jon Kleinberg, Eva Tardos. Pearson Education, 2006.
- Computer vision: Algorithms and applications Richard Szeliski. Springer, 2010.
- Machine learning: a probabilistic perspective Kevin P. Murphy. MIT Press, 2012.
- Convex optimization Boyd, Stephen P., and Lieven Vandenberghe. Cambridge university press, 2004.
- Probabilistic graphical models: principles and techniques Koller, Daphne, and Nir Friedman. MIT press, 2009.
Exercises
Exercise class: Tuesday, April 7-11 2014, 13:30-18:00 also in the HCI, 2nd floor, Speyerer Str. 6
Teaching Assistant: Christoph Straehle
Exercise 0 - Introduction to python
Some links to relevant introductions to python. We provide a virtualbox disk image with an installation of ubuntu and all dependencies neccessary for the exercises:Exercise 1 - Stereo cost volume and filtering
- Input images: scene1.row3.col1.png, scene1.row3.col3.png
- Exercise sheet
Exercise 2 - OpenGM
- Input cost volume: costvol.h5
- Python skeleton code: potts_model.py
- Exercise sheet
Exercise 3 - Dynamic programming
- Segmented least squares. Information can be found in the slides on Dynamic Programming I from Kleinberg and Tardos.
- Exercise sheet